Social interactions happen in many forms. In particular, when dealing with multi-person scenarios, the presence of competitive or cooperative interactions is not uncommon. Adapting artificial agents, whether embodied robots, or virtual avatars, to perform complex social interactions means we must take into consideration competitive and/or cooperative characteristics. Artificial agents with the ability to deal with these characteristics are still not a reality, although several researchers focused on solving these problems in the last decades based on the individual perspectives of Human-Robot Interaction (HRI) or machine learning. Recently, we did see substantial advancements in the understanding of social signals, in the modeling of multi-agent interaction, and in the development of embodied and intelligent avatars.
Yet, these are often confined to individual approaches, such as reinforcement learning or wizard-of-oz interactions, or constrained environments, for instance virtual simulations or lab-only contexts. As a result we do not see the embracing of these technologies in the mainstream solutions for real-world human-computer and human-robot interaction. Also, most of the research in these areas does not take into consideration all the technological, social, and ethical impacts of such applications. In this workshop, we put together the top-tier researchers on machine learning, in particular social signal processing, and human-robot interaction to discuss, understand and propose possible solutions for our overall topic “Competition and Cooperation: How to integrate machine learning and human-robot interaction in meaningful applications?”. Our intention is to leverage a enriching discussion of the existing problems on competitive and cooperative interactions on the multidisciplinary view of our contributors, and not to present individual solutions for each of the related topics. Our invited speakers, all experts in their respective areas, will discuss the open problems involving social agents interactions within their specialty. We also invite the submissions of position papers that present a critical view on this topic to complement and widen our discussions.
Workshop Research Topics
Topics of interest include, but are not limited to:
- Supervised, Unsupervised, and Self-supervised models for social signal processing.
- Reinforcement Learning for Multi-agent interaction.
- Representation Learning and Decision Making applied for
- Competitive and/or Cooperative Scenarios.
- Human-Robot interactions in Real-world Competitive and/or Cooperative scenarios.
- Ethical aspects of artificial agents and social interactions.
- Affective Computing Models and Applications for Multi-Agent Interactions.
- Cognitive Models for Social Interaction.
- Lifelong and Continual Learning for Interactions with Humans.
- Learning with Humans in the Loop.
- Developmental Learning for Social Signal Processing.
- Explainable Social Agents.
- Social Impact of novel Developments on Artificial Agents and Social Interactions.
- Cognitive models for Social Interaction.
Program
The final program will be updated here in the next few days.
Date: TBD
- Welcome by the Organizers (10min)
- Invited Speaker 1 (30min)
- Invited Speaker 2 (30min)
- Break (10min)
- Invited Speaker 3 (30min)
- Invited Speaker 4 (30min)
- Contribution Papers Spotlight (20 min)
- Poster Session and Lunch Break (120 min)
- Invited Speaker 5 (30min)
- Invited Speaker 6 (30min)
- Discussion Panel (30min)
Calls
The workshop holds a public call for short (up to 4 pages) and long papers (up to 8 pages) that address any of the related topics. We encourage the participants to submit not only contribution papers but also position arguments about the current problems involving any of the related topics. The authors should follow the IJCAI paper template.
We will enforce a single-blind review process and we will guarantee that each paper will receive at least two independent reviewers. Each paper can be followed by supplementary materials. The accepted papers will be published on a series related to the workshop at the Proceedings of Machine Learning Research (PLMR).
Preliminary Dates:
Paper submission deadline: May 14, 2021
Decisions: May 21, 2021
Camera-ready papers due: June 14, 2020
Submission website: https://cmt3.research.microsoft.com/CompCoop2021
Chef's Hat Competition
Most of the current Reinforcement-Learning solutions, although having real-world-inspired scenarios, focus on a direct space-action-reward mapping between the agent's actions and the environment’s state. That translates to agents that can adapt to dynamic scenarios, but, when applied to competitive and/or cooperative cases, fail to assess and deal with the impact of their opponents. In most cases, when these agents choose an action, they do not take into consideration how other agents can affect the state of the scenario. In competitive scenarios, the agents have to learn decisions that a) maximize their chances of winning the game, and b) minimize their adversaries' goals, while in cooperative scenarios b) is inverted. Besides dealing with complex scenarios, such solutions would have to deal with the dynamics between the agents themselves. In this regard, social reinforcement learning is still behind the mainstream applications and demonstrations of the last years.
We recently introduced a card game scenario for reinforcement learning, named Chef’s Hat, which contains specific mechanics that allow complex dynamics between the players to be used in the development of a winning game strategy. A card game scenario allows us to have a naturally-constrained environment and yet obtain responses that are the same as the real-world counterpart application. Chef’s Hat implements game and interaction mechanics that make it easier to be transferred between the real-world scenario and the virtual environment.
Our challenge will be based on Chef’s Hat and will be separated into two tracks: a competitive and a cooperative scenario. In the first track, the participants will use the already available simulation environment to develop the most effective agents to play the Chef’s Hat card game and be the winner. In the second track, they will have to develop an agent that can increase the chances of a dummy agent winning the game.
Challenge Organization
Each participant has to produce up to five agents that have learned how to play the game. For each track, the winner will be chosen based on the track’s specific goal. For both tracks, each competitor will pass through the following process:
- Validation: Each participant’s agent has to pass the baseline test. It will play a single 15 points game against three baseline competitors. The agent has to win the game to be eligible for the next step.
- Track 1: The agents who pass the validation step will be organized in a competition. Brackets of 4 players will be randomly drawn and separated into a competition cup scenario. For each bracket, the two best agents will pass to the next phase. The agent who finishes the championship in the first position will be crowned the winner of the first track.
- Track 2: The agents who pass the validation step will be organized in a competition. Brackets of 4 players, composed of 2 competitors agents and 2 dummy agents will be randomly drawn and separated into a competition cup scenario. Each competitor agent will be associated with one dummy agent. The two best players of each bracket will advance to the next competition phase. The agent who manages to reach the furthest, together with its associated dummy agent, will be crowned the winner of track 2.
To participate on the compeition: https://www.whisperproject.eu/chefshat#competition
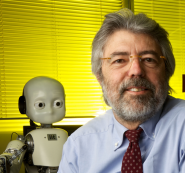
Giulio Sandini
Giulio Sandini is a Founding Director of the Italian Institute of Technology where in 2006 he established the department of Robotics, Brain, and Cognitive Sciences. He has been a full professor of bioengineering at the University of Genoa, assistant professor at the Scuola Normale Superiore in Pisa, and Visiting Research Associate at the Department of Neurology of the Harvard Medical School. In 1990 he founded the LIRA-Lab (Laboratory for Integrated Advanced Robotics, www.liralab.it) and in 1996 he was Visiting Scientist at the Artificial Intelligence Lab of MIT.
Since 1980 Giulio Sandini coordinated several international projects in the area of computer vision, cognitive sciences, and robotics. Among them the project RobotCub, funded by the "Cognitive Systems" unit of the European Union from 2004 to 2010, where he coordinated the activities of 11 European partners contributing to the realization of the iCub humanoid platform as a tool to investigate human sensory, motor, and cognitive development. (FP6-P004370: https://cordis.europa.eu/project/rcn/71867/factsheet/en).
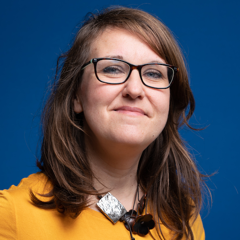
Nicoletta Noceti
Nicoletta Noceti is an assistant professor in Computer Science at DIBRIS, University of Genoa,
where she has been previously a research associate (2010-2017). She is one of the founding
members of the Machine Learning Genoa (MaLGa) center where she is co-PI of the Machine
Learning and Vision research unit.
Her research activity is focused on Computer Vision and Machine Learning for the general goal of image and video understanding. In the last years, her main interests have been on
human motion understanding problems, with applications to human-machine interaction,
robotics, video-surveillance, and Ambient Assistive Living.
She authored more than 60 peer-reviewed papers on international conferences and top-quality journals,
and she has been involved in national and international research projects and technology transfer and development programs with SMEs and large companies.
(https://ml.unige.it)
Vincenzo Lomonaco
Vincenzo Lomonaco is an Assistant Professor at the University of Pisa, Italy, and President of ContinualAI, a non-profit research organization and the largest open community on Continual Learning for AI. Currently, he is also a Co-founder and Board Member of AI for People. His main research interests include open science and ethical AI developments, continual/lifelong learning with deep architectures, multi-task learning, knowledge distillation, and transfer, and their applications to embedded systems, robotics, and internet-of-things. (https://www.vincenzolomonaco.com/)
Angelica Lim
is an Assistant Professor of Professional Practice in Computing Science at Simon Fraser University. Her research interests include human-robot interaction, including social and affective robotics, machine learning for multimodal perception and intrinsic models for social agents. ( http://www.angelicalim.com/ )
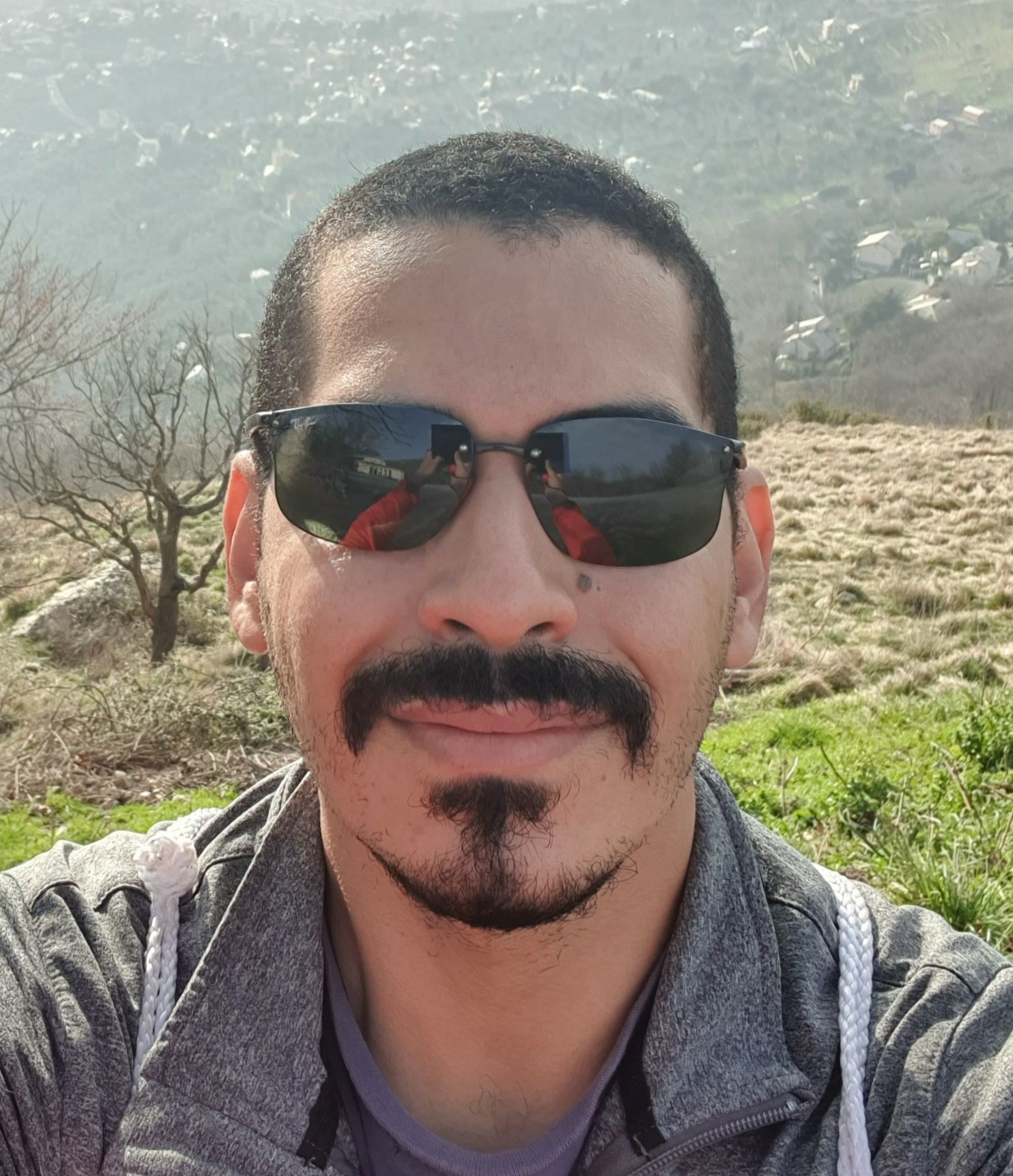
Pablo Barros
Pablo Barros was the main organizer of the Workshop on Computational Models for Crossmodal Learning (CMCL) 2017 at the Joint IEEE International Conference on Development and Learning and Epigenetic Robotics ICDL-EPIRO, Lisbon, Portugal, 2017; the main organizer of the Workshop on Intelligent Assistive Computing at IEEE World Congress on Computational Intelligence and theOMG-Emotion Recognition Challenge both at the WCCI/IJCNN, Rio de Janeiro, Brazil, 2018; the main organizer of the Workshop on Crossmodal Learning for Intelligent Robots at the IEEE International Conference on Intelligent Robots and Systems IROS, Madrid, Spain, 2018; the main organizer of the OMG-Empathy Workshop and Challenge at the IEEE International Conference on Automatic Face and Gesture Recognition FG, Lille, France, 2019; the main organizer of the Workshop on Affective Shared Perception at the IEEE International Conference on Development and Learning and Epigenetic Robotics ICDL-EPIRO, Valparaiso (virtual), Chile, 2020; and Currently, he is the Publication Chair of the IEEE International Conference on Development and Learning and Epigenetic Robotics ICDL-EpiRob 2020, Valparaiso (virtual), Chile. He received his Ph.D. degree in computer science from the University of Hamburg, Germany. His research involves affective computing and affective robots, in particular on the development of deep and self-supervised deep neural networks. Currently, he is a research scientist at the Italian Institute of Technology, Italy.
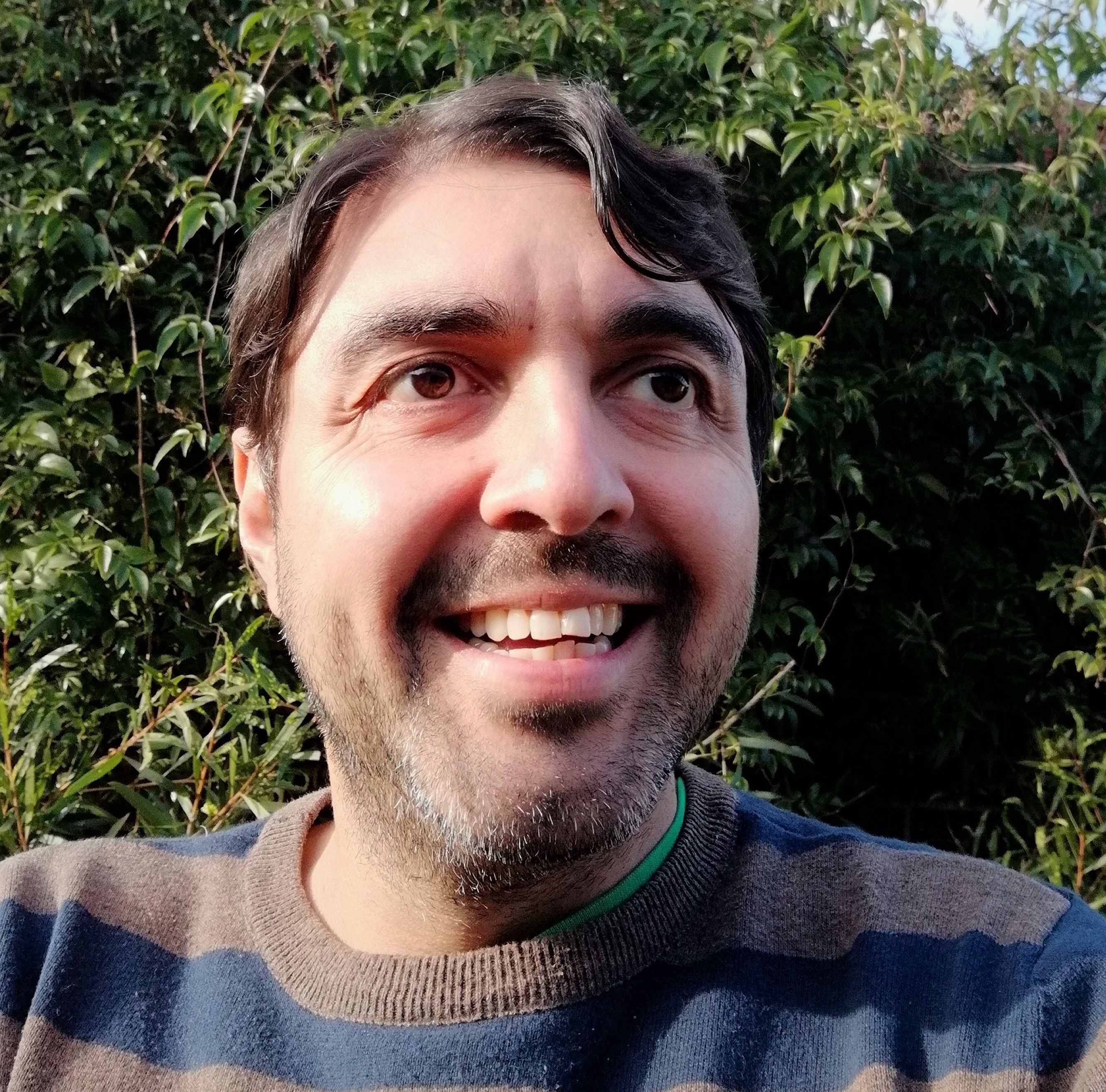
Francisco Cruz
Francisco Cruz received the bachelor’s degree in engineering and the master’s degree in computer engineering from the University of Santiago, Chile, in 2004 and 2006, respectively. In 2017 he received his PhD degree from the University of Hamburg, Germany working in developmental robotics focused on interactive reinforcement learning. In 2015 he was a visiting researcher at the Emergent Robotics Laboratory at Osaka University. He joined the Engineering School at Universidad Central, Chile as a Lecturer in 2017 and the School of IT at Deakin University as a Research Fellow in 2019. He has been on the organizing committee of international conferences and workshops such as ICDL-EpiRob 2020, the Workshop on Bio-inspired Social Robot Learning in Home Scenarios at IROS 2016, and the Workshop on Intelligent Assistive Computing at WCCI/IJCNN 2018. He has served as a reviewer of several international journals and conferences. In 2017, he was a Guest Editor for the journal IEEE Transactions on Cognitive and Developmental Systems (TCDS). He is currently an Associate Editor for contributed papers of the IEEE ICDL-EpiRob conference. His current research interests include reinforcement learning, explainable artificial intelligence, human-robot interaction, artificial neural networks, and psychologically and bio-inspired models.
German I. Parisi
is the Director of Applied AI at McD Tech Labs in Mountain View, California, a Silicon Valley-based research centre established by McDonald’s Corporation. He is also an independent research fellow of the University of Hamburg, Germany, and the co-founder and board member of ContinualAI. He received his Bachelor's and Master's degree in Computer Science from the University of Milano-Bicocca, Italy. In 2017 he received his PhD in Computer Science from the University of Hamburg on the topic of multimodal neural representations with deep recurrent networks. In 2015 he was a visiting researcher at the Cognitive Neuro-Robotics Lab of the Korea Advanced Institute of Science and Technology (KAIST), South Korea, winners of the 2015 DARPA Robotics Challenge. He was the co-organizer of the Workshop on Lifelong Learning for Long-term Human-Robot Interaction at IEEE RO-MAN 2020 in Naples, Italy, the Workshop on Continual Learning in Computer Vision at CVPR 2020 in Seattle, WA, the Workshop on Crossmodal Learning for Intelligent Robotics at IEEE/RSJ International Conference on Intelligent Robots and Systems (IROS)'18 in Madrid, Spain, the Workshop on Intelligent Assistive Systems at IEEE World Congress on Computational Intelligence (WCCI-IJCNN) '18 in Rio de Janeiro, Brazil, and main organizer of the Workshop on Computational Models for Crossmodal Learning at the IEEE International Conference on Development and Learning and on Epigenetic Robotics (ICDL-EPIROB) '17 in Lisbon, Portugal.
Doreen Jirak
was member of local organizers of the International Conference on Artificial Neural Networks, ICANN, Hamburg, Germany, 2014 and sole organizer of a special session on Human-Computer Interaction, funded by German Koerber Foundation for Young Researchers; the main organizer of the Workshop on Computational Models for Crossmodal Learning (CMCL) 2017 at the Joint IEEE International Conference on Development and Learning and Epigenetic Robotics ICDL-EPIRO, Lisbon, Portugal, 2017; the main organizer of the Workshop on Crossmodal Learning for Intelligent Robots at the IEEE International Conference on Intelligent Robots and Systems IROS, Madrid, Spain, 2018; She received her PhD degree in computer science from the University of Hamburg, Germany. Her research is centered around the understanding of artificial neural networks, especially the paradigm of “Reservoir Computing”, on nonverbal communication with special focus on gesture recognition. The overarching goals of her research are to link principles of learning to human-robot interaction (HRI) as an contribution to the development of natural HRI interactions and social robots. Additionally, Doreen Jirak serves as reviewer for several important conferences and journals (e.g. HRI; IROS; Neural Networks). She has 10 years of experience in successfully organizing and holding seminars and lectures in the relevant fields like “Machine Learning for HRI”, “Artificial Intelligence”, “Knowledge Processing in Intelligent Systems”, and “Data Mining”.